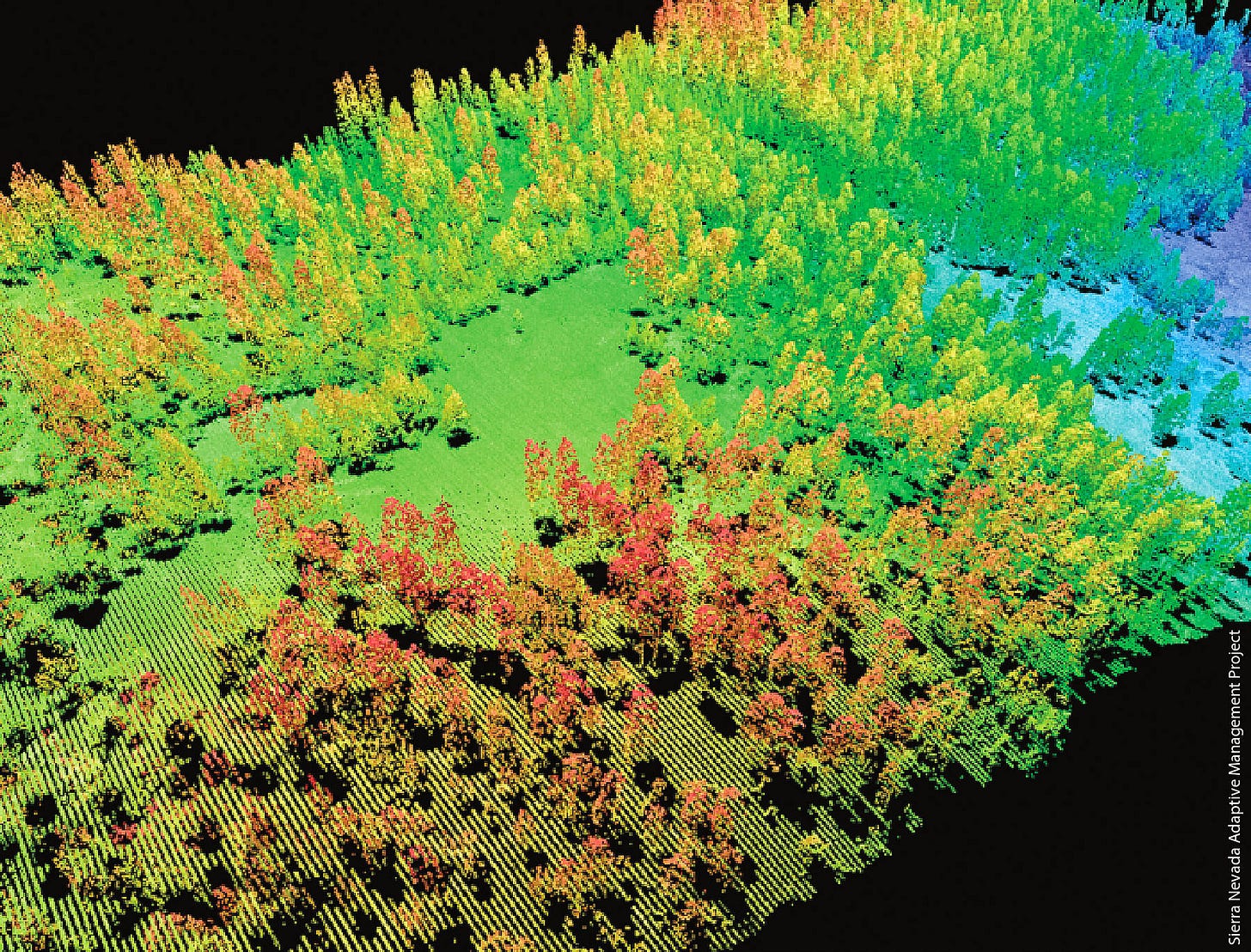
The use of remote sensing technologies and GIS have been the primary practice for providing insights to large hectares of forested land due to its high spatial and temporal coverage allowing for wall-wall monitoring, mapping and managing forests. Data products from these are derived from spaceborne, airborne and even from terrestrial platforms, and due to its unique vantage points, provides a complete survey of the environment that otherwise would have been difficult if using conventional
field survey methods that are based on sparse sampled points for interpolation over the study area.
Remote sensing imagery from spaceborne earth observation (EO) satellites come in various spatial and temporal scales. The LandSat legacy has been collecting imagery of earth for over 50 years, providing a rich database of temporal landcover change and it was not until 2008 that the United States Geological Survey (USGS) made it freely available. This data policy change led to useful applications and insights for research in many segments of society in mapping annual global forest change from 2000 – 2012 which was found to accrue a net forest loss of 1.5 million km2. Landsat imagery however comes in coarser resolutions from 30-120m. Despite that, there are a plethora of satellite sensors such as sentinel 1 and 2 with spatial resolutions at 10 – 60m and Worldview-1 and 2, which are commercially available at much higher resolutions. Airborne remote sensing also produces much higher resolution imagery at 20cm however requires some investment in conducting the survey mission.
LiDAR provides by far the most detailed survey especially in precision forestry. It’s ability to penetrate tree canopy and foliage and providing forest structure from point clouds that optical imagery lacks make its use highly invaluable to studies looking into forest metrics used for biomass. The system can be either full waveform or as discrete return recordings. Full waveform LiDAR systems allow for a complete capture of the forest structure as it profiles the vegetation structure such as branches, stems, leaves and the ground from one return. Its footprint is a circular illumination of the ground and can be larger than 10m in diameter whilst discrete returns usually have smaller footprints down to sub-centimeter levels. Typical products derived from this sensor are digital elevation and surface models.
The Global Ecosystem Dynamics Investigation (GEDI) mounted aboard the International Space Station (ISS) provides the highest resolution and dense sampling density from its environment in space and it is freely accessible via its website.
LiDAR sensors consist of 3 main components: a laser beam for measuring the surface cover; an inertial measurement unit that records the orientation on its axis; and an on-board GPS or GNSS for position measurements. The platform can be mounted on an aircraft or a UAV and works by emitting a pulsed light beam onto the study region of interest under the sensor. These three components function synchronously to capture the return times and locations when the beam bounces back of these surfaces. The wave forms are able to measure and quantify the vertical structure of vegetation by
recording the amount of laser energy reflected from stems, branches and leaves and records this as a point in 3D space known as point clouds. Besides DEM and DSM, other products derived from lidar for forestry related studies include canopy height metrics, canopy cover metrics, and vertical structure metrics.
These sensors alone cannot make AGB estimates directly and so would need a sufficient enough in-situ measurements from forest plots along with allometric models to develop accurate AGB estimates and evaluation results, and bridging this is a GIS. Being a platform of hosting spatial databases, it synthesizes remote sensing and field data by integrating georeferenced data for a comprehensive and integrated spatial analysis. An earlier study conducted by Brown (1997) had used GIS modelling to predict biomass change for tropical forests using several GIS data layers which included, soils, precipitation, vegetation, national and sub national boundaries
and topography maps along with population estimates and forest inventory, highlighting the importance and feasibility of the role of GIS modelling in AGB change estimation.